Retaining customers is a top-line priority for any marketer. Increasingly companies are proactively* implementing strategies aimed at persuading customers to stay. A pivotal question for the success of a retention strategy is who to select? Which customer is at risk of leaving the company and how many of them should be included in a campaign. Being smart about selection can mean the difference between success and failure. Below I describe three data-driven approaches to select customers for your retention strategy.
Approach 1: Churn Probability
Using historic retention/churn data in their retention strategy, companies can make predictions about which customers are most likely to leave. Based on the distribution of this churn probability, a company could make a selection, say the top 25% of customers who have the highest probability of leaving. This churn-driven method has been the go-to approach over the past years, but it ignores a crucial factor: sensitivity towards the offer. Customers with the highest probability of leaving, are not necessarily the ones most receptive to an offer. Consider this often used 2 X 2 to put this in perspective.
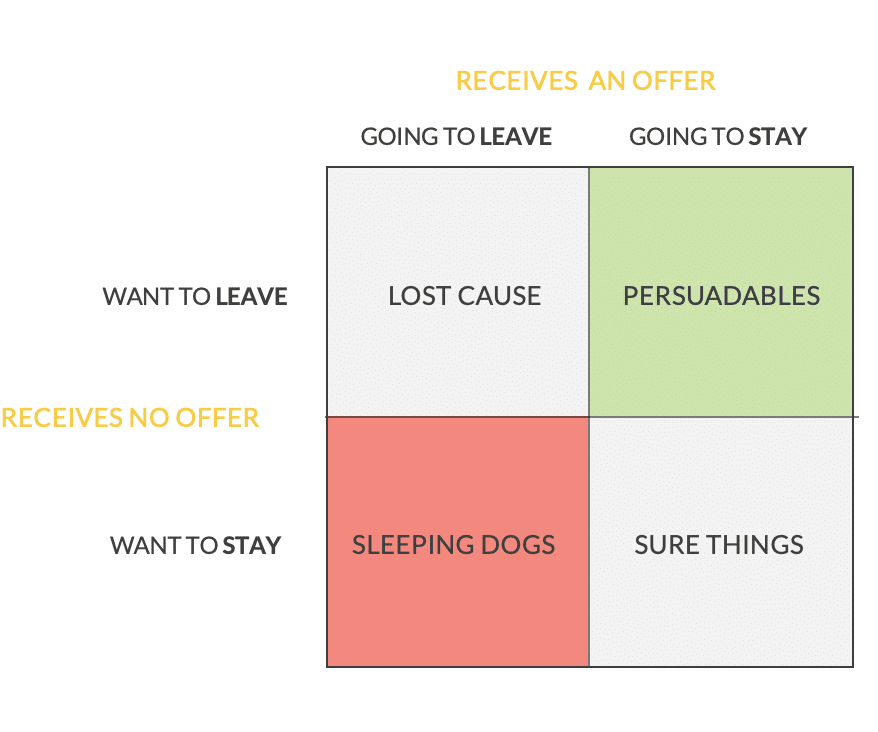
The matrix describes the treatment (receiving an offer) and the response (staying or leaving). Two things to notice; first, there are two groups where the campaign has no effect (sure things and lost cause), in which case resources are waisted as the customer behavior is not altered. Customers who were going to leave do, and those who wanted to stay do so as well, with or without an offer. Second, such strategies may have a positive or negative effect on the customer’s decision to stay with the company. Negative effects, occur if an offer is made which prompts a customer to leave, which otherwise would have stayed (sleeping dogs). Positive effects (persuadables) are the ones, marketers should go after in their retention strategy.
A churn based approach does not explicitly focus on the persuadables, leading to a suboptimal or even harmful outcome. There must be a better way, and there is.
Approach 2: Uplift
A way to address this problem is to run an experiment and use the outcome to assess which customer to include. It works like this, a small experiment ( e.g. A/B test ) is run where one sample of customers receives a retention offer and a control group does not. Based on this data a model is trained to predict the additional (uplift) number of customers that will stay because of this campaign (Ascarza 2018). Uplift models are a technique that directly models the incremental impact of a treatment (such as a retention offer) on an individual’s behavior. The uplift is the probability of customers who stay and receive an offer minus the probability of customers who stay and did not receive an offer. For example, if 55% of the customers who received an offer stayed, while 50% of the customers who did not receive an offer stayed, our uplift would be 55% – 50% = 5 percentage points.
Furthermore, the model provides an importance score in terms of what are the main features of customers are associated with customers who change stay (e.g. demographics, purchase history, location, etc.). Using this model trained on a small sample of the customer base, the rest of the customers who were not part of the experiment can now be ranked according to their propensity to stay in case they receive an offer.
The great thing about uplift models is that a causal link can be established between the campaign and the change in customer behavior. Investment in running such an experiment is limited, and also provides an opportunity to test different incentives to see which works best. In doing so, you now have a data-driven way to rank customers by how sensitive they are to a retention offer. Great, but can we do even better? Yes.
Approach 3: Profit based lift
Not all persuadables are equal, however. Customers represent different value to the company. Some customers may be open to staying with the company but are not “worth” having. Using customer lifetime value (e.g. defined as the discounted future cash flows of an individual customer) the selection of customers to retain can be further optimized (Lemmens and Gupta et al 2020). Incorporating CLV and the cost of the campaign provides a long term and profit-based approach to selecting customers who are not only likely to change their behavior but also represent the most value for the company. In essence, the approach is the same, a A/B test is conducted but this time we include CLV as a data point which is used to find the optimal (most euro’s) outcome.
Recent research (Lemmens and Gupta 2020) applies this approach to a European TV subscription service and the researchers find selecting customers based on a profit lift function deliver 21% more value than using a churn rate-based approach. So just by altering the way the customers were selected value is generated by the same campaign, resulting in a boost of overall revenues by 3% for this particular company.
Conclusion
Retention of customers has always been a top priority for marketers, with budgets being tight, this might be even more true today. If you are thinking about a retention strategy, be smart about your selection. Run an experiment to find persuadables and if possible use CLV to decide who you really want to retain. By implementing these analytics, you can not only keep customers, but also improve the overall bottom line. Two things that should make any marketer smile.
If you want to find out more and see some live cases, consider joining our webinar on customer analytics on the 26th of May. Join the webinar here.
Footnotes
* Pro-active refers to customer who have not indicated they are leaving yet.
** The intention (wanting) is normally not part of this distinction but it makes the matrix easier to comprehend in my view.
References
Ascarza, Eva (2018), “Retention Futility: Targeting High-Risk Customers Might Be Ineffective,” JMR, Journal of Marketing Research, 55, 1, SAGE Publications Inc, 80–98, doi:10.1509/jmr.16.0163.
Lemmens, Aurelie and Sunil Gupta (2020), “Managing Churn to Maximize Profits,” Marketing Science, Forthcoming, doi:10.2139/ssrn.2964906.
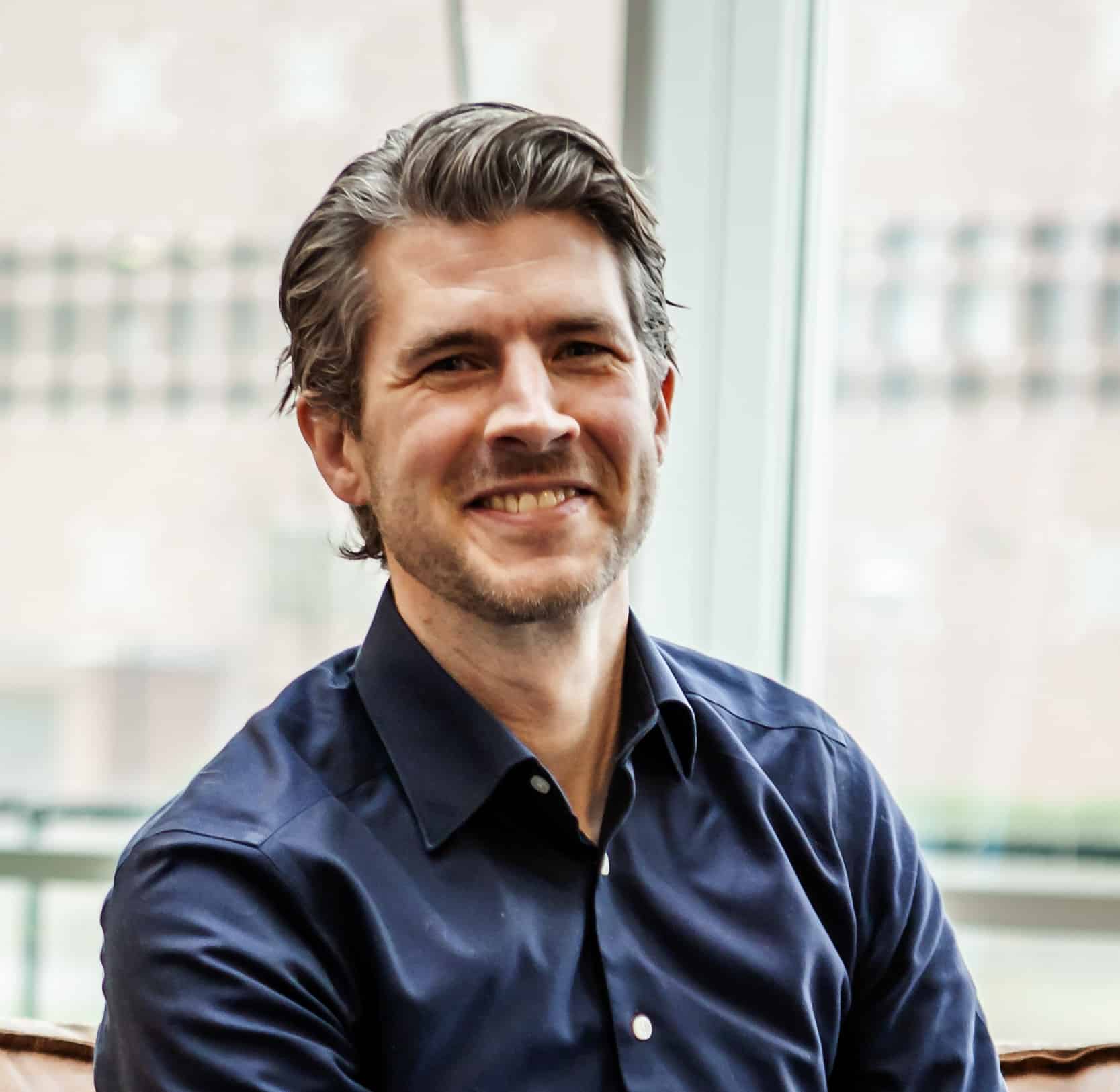
Bob Rietveld
Head of Growth Analytics
Related posts
5 Growth Mindset Examples That You Can Use in Your Job Tomorrow
People who believe their success is based on hard work,…
September 15, 2022
Who are my most valuable customers?
Legend has it Alfredo Pareto noticed something unusual in…
April 22, 2020
Why customer lifetime value analysis is now more important than ever
Over the course of the last weeks we, at RevelX, have been…
April 22, 2020